Progress Report
The Realization of an Avatar-Symbiotic Society where Everyone can Perform Active Roles without Constraint3. Human level knowledge and concept acquisition
Progress until FY2022
1. Outline of the project
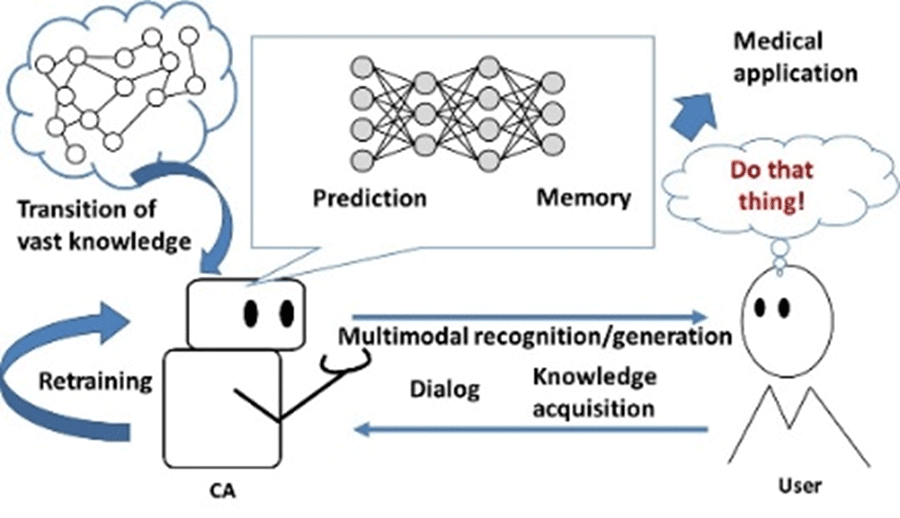
In this project, we work on the acquisition of human knowledge and concepts necessary for advanced cognitive abilities, knowledge sharing among cybernetic avatars (CAs), and understanding of the operator's intentions to realize semi-autonomous functions for cybernetic avatars (CAs) that move according to the user's intentions.
This knowledge and concept acquisition requires combining visual information with human-level knowledge and concepts and information from different modalities, including natural language. We also aim for CAs to acquire knowledge and concepts that can be shared with humans through the fusion of different modalities, such as visual information and natural language, and the realization of dialogues for knowledge sharing and acquisition in various real-world environments. Furthermore, observations and dialogues based on the acquired knowledge and concepts are used to understand the intentions of operators and users. Since it is difficult to control the environment for interactions between CA, operators, and users, and since the amount of data obtained is small, we will develop a new method for learning with a small amount of data to acquire new knowledge and concepts.
2. Outcome so far
In order for CA to share knowledge and interact with humans, it is essential to understand not only the real world semantically, but also the underlying geometry. In particular, geometrical understanding requires spatio-temporal modelling, including non-rigid objects that change shape over time. The object deforms drastically from moment to moment, and the point cloud representing the observed appearance and depth varies greatly, even if the object is the same. To solve this problem, we worked on matching and registration problems between point clouds of partial observations with large deformations and a free-form deformation method for radiance fields.
For partial point cloud matching in rigid and deformed scenes, we propose a learning-based method. The features of our method are a position encoding technique that separates the point cloud representation into a feature space and a three-dimensional position space to represent relative distance information, and a repositioning technique that changes the relative positions between point clouds. Our proposed method achieves twice the reproducibility of non-rigid feature matching compared to previous studies on a particularly challenging dataset for non-rigid point cloud matching. Our work has been accepted for oral presentation at CVPR2022.

In addition to partial point cloud matching, a study of non-rigid point cloud registration was also conducted. Non-rigid point cloud registration is challenging due to the high complexity of the unknown non-rigid motion. In this study, we solved this problem by using hierarchical motion decomposition. The hierarchical motion representation allows a multi-step decomposition from rigid-body to non-rigid-body motion, which leads to higher accuracy and speeds up the solution by a factor of 50 compared to existing neural network-based approaches. The work has been accepted for NeurIPS2022.
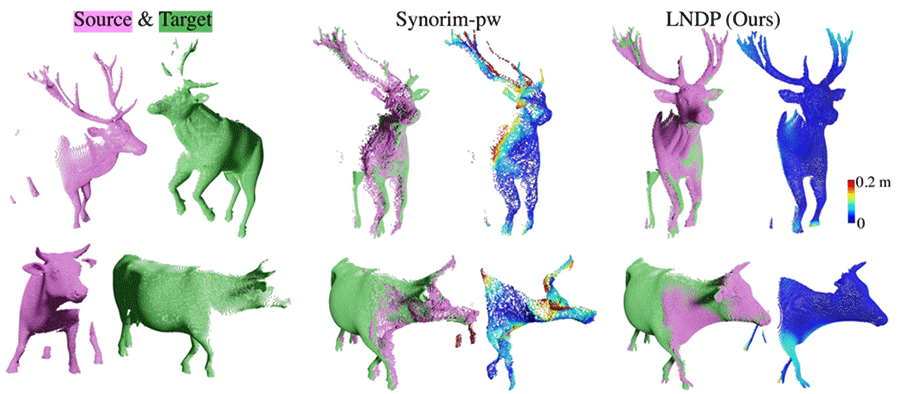
Furthermore, we proposed a new method to deform the radiance field: free-form deformation of the luminance field. This method uses a triangular mesh surrounding a foreground object, called a cage, as an interface and allows free-form deformation of the radiance field by manipulating the vertices of the cage. This work has been accepted for ECCV2022.
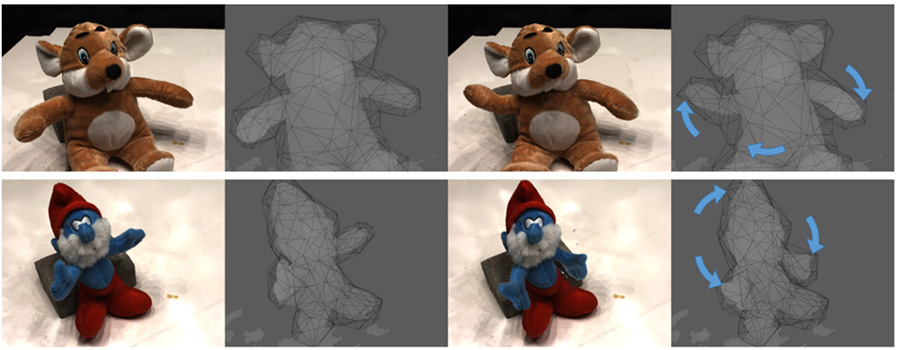
3. Future plans
The challenge will be to advance and integrate each element in the future.