Progress Report
Artificial generation of upstream maritime heavy rains to govern intense-rain-induced disasters over land (AMAGOI)[1] Control Theory
Progress until FY2023
1. Outline of the project
There are three hurdles in manipulating weather. First, we need to find effective interventions. Careful selection of interventions is necessary, as an ill-planned intervention may not necessarily produce the desired outcome. Secondly, interventions must be feasible. Weather, being large-scale, can require enormous amounts of energy to control, while there are also constraints on the amount of energy we humans can expend. Finally, the intervention must be computable; vercoming the large-scale nature of weather phenomena, interventions must be computed in the short time between when heavy rainfall damage is predicted and when it occurs. Althouhg the control theory can be essential to overcome these three hurdles, the current control theory has not dealt with such large-scale phenomena.
To clear these hurdles, two research tasks have been established in our item. Subject 1-1 Development of Feedback Control Theory develops the concept of feedback control and constructs a control theory that can overcome the above hurdles. To complement this subject, Subject 1-2 Development of Data-driven Control Theory develops weather control methods that transcend the framework of existing control theory by utilizing data technologies such as ensemble prediction in addition to AI techniques. Through the promotion of these subjects, our item will demonstrate the feasibility of mitigating heavy rainfall over land through offshore rainfall generation on a computational basis.

2. Outcome so far
- [Model Selection (1-1)]
- The development and evaluation of control methods require an easy-to-handle mathematical model capturing the essence of meteorological phenomena. The Lorenz model has been used in the past but is limited in scale. We choose to use Ideal Atmospheric Experiment (right figure) available in the SCALE Regional Model. This model's features are expected to significantly accelerate weather control research
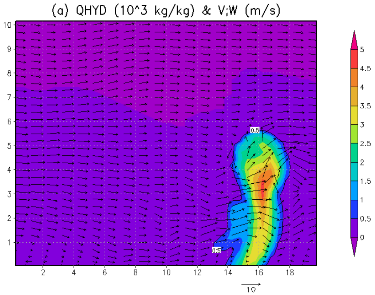
- [Evaluation of Existing Control Methods (1-2)]
- To establish a solid research foundation, we evaluated the performance of an existing weather control method [Miyoshi and Sun, 2022]. This method is known to struggle to find effective interventions depending on the forecast interval size. By comparing its performance with Model Predictive Control, we confirmed that this issue stems from the limitations of the traditional method.
- [Development of Data-Driven Methods (1-2)]
- In the above evaluation, we incorporated a technique called deep unfolding into Model Predictive Control (see figure below). Deep unfolding is a machine learning technique widely used in developing Beyond 5G wireless communication algorithms. By integrating deep unfolding, we effectively optimized interventions in Model Predictive Control.
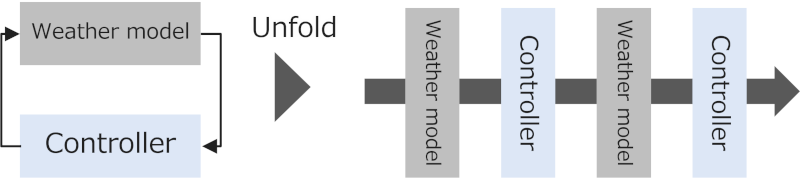
- [Theoretical Development to Overcome Large-Scale Challenges (1-1)]
- Meteorological phenomena are large-scale, making the direct application of traditional feedback control methods from control theory computationally challenging. In this study, we developed an intervention calculation method using a fast optimization framework called convex optimization. In this direction, by preparing perturbation analysis in advance, we enabled interventions to be computed within a few seconds, even in the Ideal Atmospheric Experiments accompanied with thousands of state dimensions. Simulations confirmed that the obtained interventions are indeed effective (see right figure).
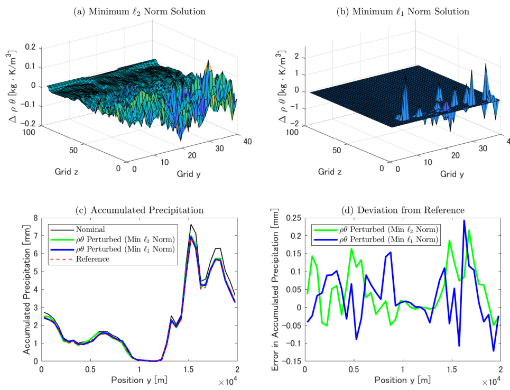
3. Future plans
Building on the above technological developments, we will further continue our research to achieve our 2024 milestone of reducing precipitation by 10% in Ideal Atmospheric Experiments. For Subject 1-1, we will extend the current method to calculate continuous feedback interventions and conduct comprehensive evaluations in the Ideal Atmospheric Experiments. For Subject 1-2, besides Model Predictive Control using deep unfolding, we will develop intervention discovery methods based on sample-based Model Predictive Control, expected to be scalable to large-scale phenomena. To enhance the scalability of these methods, we will incorporate heuristic optimization techniques such as Bayesian optimization.