Progress Report
Integration of Asian humanities and brain informatics to enhance peace and compassion of the mind2. Neurofeedback
Progress until FY2022
1. Outline of the project
The nature of the self and the external world is an old and yet new issue that has been discussed since ancient times. In this project, we aim to develop technology for a society that coexists with machines that can observe the state transitions of the mind with the combined knowledge of Eastern thought, including Buddhism, artificial intelligence, and brain science. For this purpose, we will develop a visualization device with high temporal resolution that can adequately capture mental state transitions and use it for neurofeedback learning, aiming to learn a balance between dynamism and stability in the brain network that is not affected by the external world.
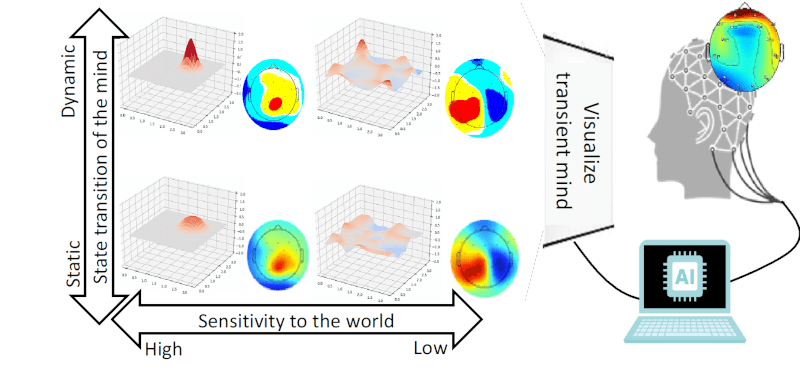
2. Outcome so far
“Visualization of brain state transitions”
EEG microstates is an EEG analysis method that has recently received renewed attention. By extracting common EEG states called templates in advance, brain state transitions can be coarse-grained as transitions between these templates. Previously, state transition networks were constructed using the four polarity-neglected templates ABCD, but in order to represent state transitions between polarity, we increased the number of templates to eight states with polarity (ABCD±).
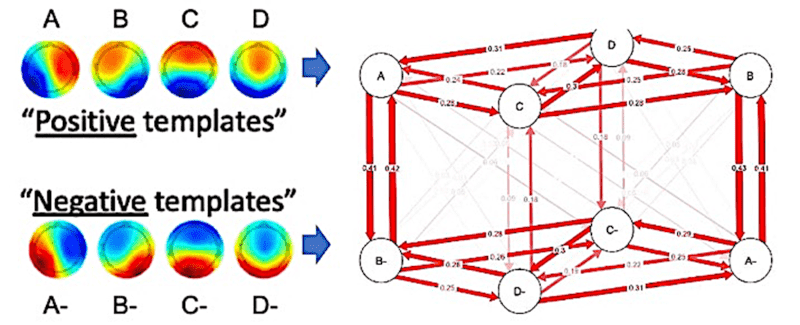
and their state transition probabilities (right)
“Feedback Technology Development”
Predefining brain states such as EEG microstates enables state detection and feedback learning in real-time. In order to capture instantaneous brain state transitions, we redesigned the neurofeedback system to achieve high temporal resolution of feedback. The system is now ready to be used to train participants in experiments to verify whether learning is successful.
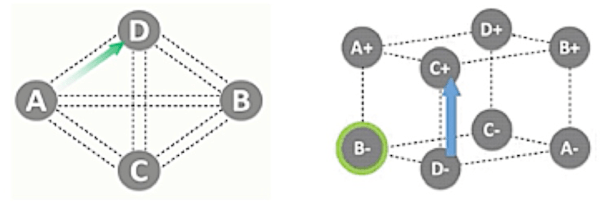
“Machine learning of brain state transitions”
By combining a generative model and a data-driven model, we confirmed that the system is designed to quantify the transitions between attractors of nonlinear dynamics in the brain, and that the data assimilation and expansion methods can be executed within an acceptable time frame. For the generative model, we will focus on the Wilson cowan model, emphasizing computational time and feasibility of parameter optimization. For the data-driven model, we will focus on the pairwise maximum entropy model (pMEM) from the viewpoint of quantification of brain state transitions.
3. Future plans
In actual neurofeedback training, too quickly visual feedback may be unusable for learning. Therefore, we plan to incorporate a gamification mechanism in which the morphing image changes depending on the hit (detection of a specified target). Ultimately, by using these technologies, we will develop technologies to realize mental peace of mind and verify the effectiveness of such technologies.
(ASAITomohisa, KAWANABE Motoaki:ATR)