Progress Report
Control Theory of Weather-Society Coupling Systems for Supporting Social Decision-Making[A-2] Uncertainty quantification for meteorological control
Progress until FY2023
1. Outline of the project
- Background:
- Research on weather control should rely on computer simulations until the safety of new intervention methods is thoroughly assessed. However, meteorological simulations inherently have uncertainties in their various modules, making it challenging to fully trust the simulation results of weather control.
- Objective:
- We will pinpoint all potential sources of uncertainties in meteorological simulations and minimize them using observation data. Then, we will quantify the residual uncertainties contributing the accurate assessment of weather control techniques.
- Method:
- We will conduct many simulations with various settings and analyze them alongside observational data using machine learning. This enables us to quantify the uncertainties of meteorological models, which have not been identified by their developers (data-driven approach). We will also comprehend the mechanisms contributing to these uncertainties (process-driven approach) (Fig.1).
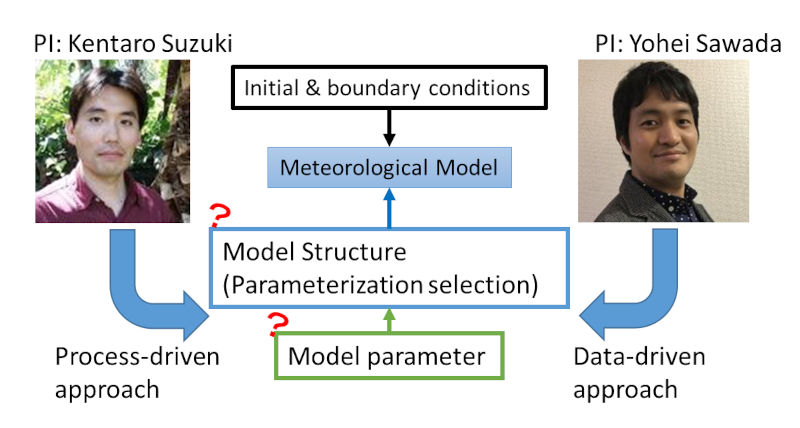
2. Outcome so far
- ①[Data-driven approach] Our novel uncertainty quantification method was successfully applied to a global atmospheric model to estimate 9216 time-varying parameters, which have been impossible in the literature. We confirmed that our method can be applied to realistic atmospheric simulation. Using this method, we successfully improve the skill to predict severe rainfall and tropical cyclone and accurately quantify their uncertainties (Fig. 2). We are also exploring the method to fully use this estimated uncertainty information for mitigating water-related disaster risks.
- ②[Process-driven approach] Cooperating with the other R&D themes, we are analyzing various large ensemble simulation data (Fig. 3). Focusing on precipitation processes, we are developing the method to interpret the uncertainties estimated by the data-driven method.
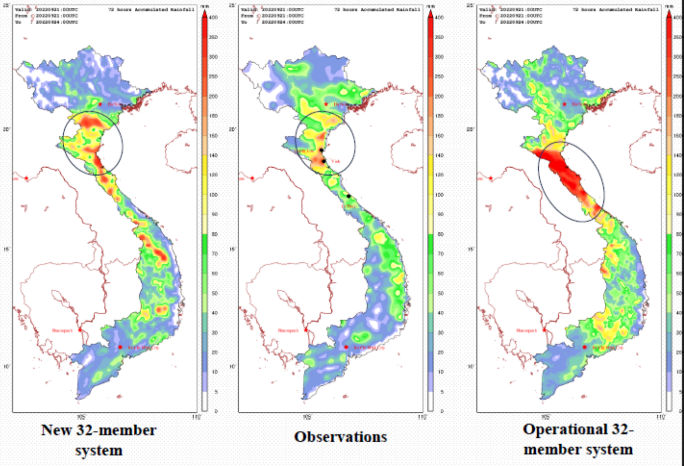

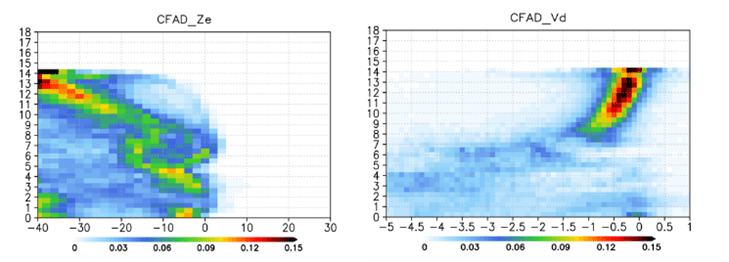
3. Future plans
The R&D of uncertainty quantification method has been completed, and the effectiveness of it has been thoroughly evaluated in the atmospheric prediction. We will explore the applied research for weather prediction and control toward discovering, quantifying, and minimizing the uncertainty in atmospheric simulation. By understanding the estimated uncertainties in the impact of weather control, we will explore “explainable” weather control.