Progress Report
Smart Robot that is Close to One Person for a Lifetime2. AIsystems for smart robots
Progress until FY2022
1. Outline of the project
Moravec's paradox is a contradiction that tasks that are easy for humans are difficult for artificial intelligence and robots. In recent years, deep learning technology has advanced rapidly, and AI and robots have been able to perform tasks such as walking, running, visual and auditory recognition, and language-based communication. However, it is still a difficult challenge for even the latest technology to perform a variety of real-world tasks using common hands and tactile sensations.
This study aims to realize robot intelligence that supports human manual labor, especially housework, by utilizing a unique approach called "deep prediction learning" based on the knowledge of neuroscience. Deep prediction learning is a framework that applies deep learning technology to predict high-dimensional changes in sensation and motion in real-time and minimize prediction error. This method has already been used to handle clothes and food and move around the house. We plan to expand the research results in the future.
2. Outcome so far
We conducted several motion learning studies using the human-collaborative robot Dry-AIREC developed in our project, as well as other robots. Here are some examples:
1)Handling various objects with a multi-fingered hand:
Object picking is fundamental for many robots. However, most research in this area is limited to grasping planning using visual information. In this study, we proposed a challenging task of grasping diverse objects using the coordinated motion of four fingers with 384 tactile sensors. We achieved this through deep predictive learning and presented our findings at IEEE RA-Letter and ICRA2022 as shown in Figure 1.
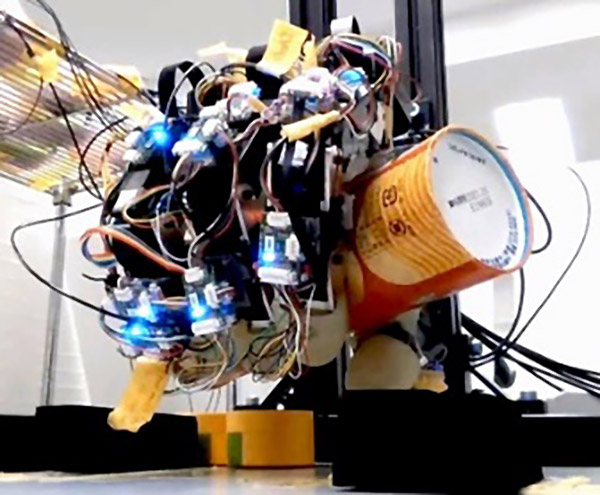
2)Laundry Task:
As part of household operations performed by Dry-AIREC, we collaborated with the Technical University of Munich to conduct laundry handling using deep predictive learning and attention mechanisms (Figure 2, left). The learning of this task was facilitated using a bilateral teleoperation device. We showcased these research findings at IEEE/RSJ IROS2022 (Figure 2, right).
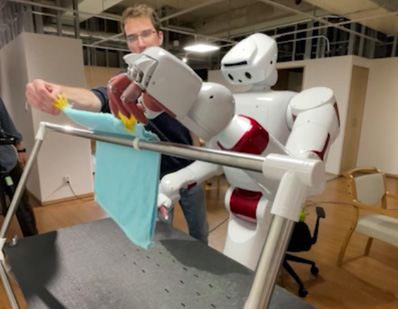
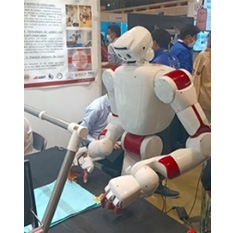
3)Language-Motion Learning:
We conducted research with the aim of integrating large language models such as ChatGPT with motion. Specifically, we developed a transformative learning model incorporating vector representations from language learning into deep predictive learning models. We presented these results at IEEE RA-L and IROS2022 and were awarded the SIYA-IROS2022.
4)Utilization of Virtual Spaces (Forcemap):
In simulated environments, it is possible to obtain information that is not visually perceivable. Taking advantage of this characteristic, we constructed a model capable of predicting force distribution for manipulating multiple objects (accepted for IROS2023). Figure 3 represents the visualization of contact force distribution obtained from multiple object images.
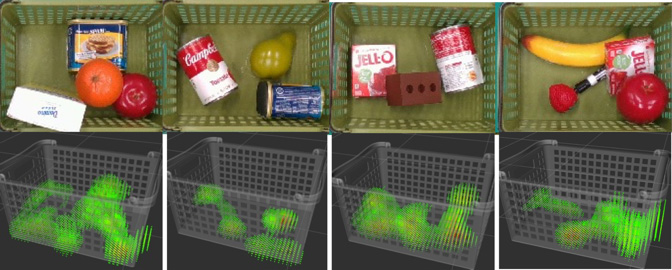
5)Model extension based on the Free Energy Principle:
We built a variation of the Bayesian recurrent neural network (PV-RNN) grounded in the theoretical framework of the free energy principle. By utilizing real robot data, we are currently advancing the updates to the existing deep predictive learning models.
3. Future plans
In the fiscal year 2023, we plan to release the open-source software EIPL (Embodied Intelligence with Deep Predictive Learning) for deep predictive learning. We will also expand the number of AIREC to enable the realization of a wider range of tasks. We will also develop real-world applications of Forcemap and extend the models through the PV-RNN framework. Furthermore, we are set to commence full-scale collaborative research with the Alan Turing Institute, UK, with whom we signed a memorandum of understanding in 2022, in partnership with Waseda University.