Using "Power Laws" to assess the degree to which AI adapts to human society
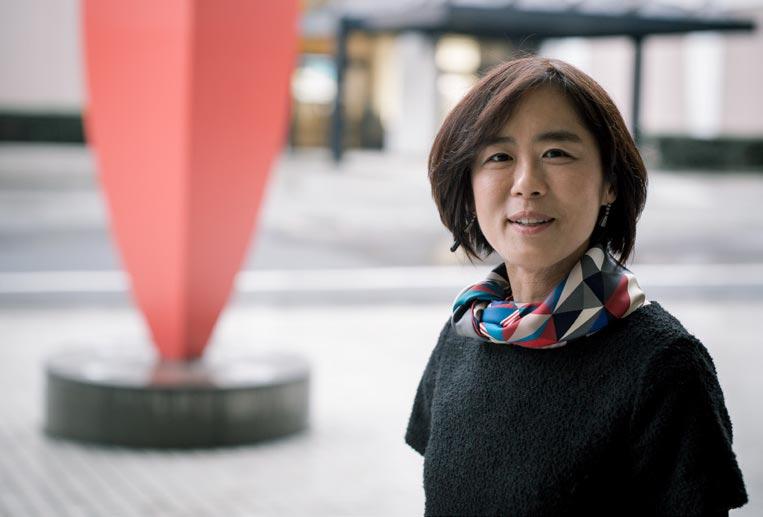
Kumiko Tanaka-Ishii
Professor of the Research Center for Advanced Science and Technology, the University of Tokyo
Area of Specialization: computational linguistics, complex systems science for natural language, communication and social systems. Principal investigator of the HITE adopted project "A co-evolutionary study on society with respect to power laws: - Can AI replicate the behavior of a non-equilibrium complex system?"
Professor Kumiko Tanaka-Ishii has been trying to comprehend the mathematical universal properties underlying human communication and natural language. She uses the perspective of a statistical model called the "power law" as the key to assessing how well AI is adapting to human society. We asked her about her research and her vision for society.
"Power laws" everywhere
What is the "power law" model?
A power law is a physical law that is observed empirically in various natural and social systems. In essence, it is a model that forms a proportional relationship between two statistical variables (excluding constant terms), when plotted on logarithmic axes.
Curiously enough, this power law distribution can be observed in various phenomena, such as earthquakes, market prices, natural language, and the population distribution of cities. For example, in earthquake statistics, plotting the energy on the horizontal axis and the frequency on the vertical axis, we see a graph that shows a clear power law distribution. Power laws can also be found between the size of cities and their population, or the number of references contained in technical papers and their frequency. Plotting the annual income of millionaires against their income ranking also produces a linear relationship.
When a graph shows a "power law", will it hold without exception?
Surprisingly enough, in most cases a power law seems to hold without exception, but this depends on the measuring technique. If the measuring technique is not good enough, a method might not show the underlying properties. I started my career studying natural language. Statistics of the words used in English texts show that the most frequent word is "the", followed by words such as "of" and "and". When the frequencies of words are plotted against their frequency rankings, a power law graph is produced. This power law has been studied to hold in various types of texts, across languages, genres, cultures, production times, authors' age and gender. It even holds with the child-directed speech of three year-olds. No one is consciously aware of talking in a way that produces a power law, but curiously enough it happens anyway. What's more, the reason for this distribution remains unknown.
How can "power laws" be utilized in the study of AI?
Machines have simple designs, so it is not clear whether a power law can be produced with machine-generated texts. For example, until a decade ago, machine-generated texts hardly show power law distribution in ways that were similar to human language. Twitter bots are designed to quote other people's words automatically, but it is still questionable whether they display a power law. Recently, however, when using deep learning, these machine-generated texts have started to display "power law"-like behavior, as shown in Fig 1. However, on the whole, AI performance has a long way to go.
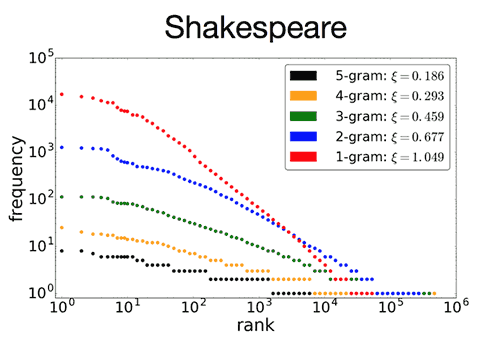
Fig 1: Power Law of the Shakespeare whole sentence.
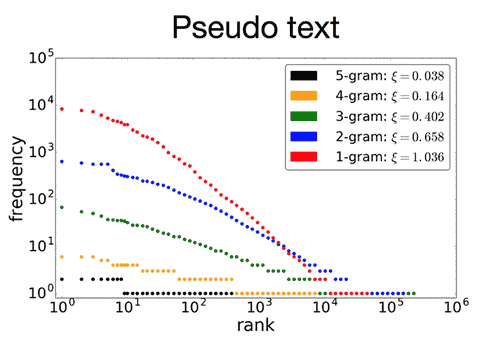
Fig 1: Power Law of the document which the deep learning that learned it generated.
This was the starting point for our project. Accordingly, we set out to consider the question "How natural are the results produced by AI?", or in other words "How well has AI adapted to human society?" using the following question as a gauge - "Do machine-generated results produce a power law?".
What is implied by the formation of a power law?
A power law presents a statistical self-similarity, similar to "fractal structures" like the Koch curves. A self-similar structure contains many similar structures but at smaller scales within the whole structure. Likewise, a power law presents the same exponents when the scale is magnified or minimized. As mentioned, this is a property that can be seen widely in nature and social phenomena, but because machines are man-made, they do not have such structures in general. Therefore, we look for power law formation as an indicator to measure whether self-similarity exists in the systems produced by machines.
Power laws as a new indicator for assessing AI
What are the merits of applying this measure to AI?
We are considering how to create a system that detects any AI systems that do not produce power laws, so that for example we could exclude AI with the potential to behave recklessly in the field of investment. In stock investments, if an AI keeps on pursuing short-sighted maximum gains, it is likely that its behavior will destroy the power law, and this could risk the whole market. We believe it might be possible to detect these signs in advance by using methods that use power laws as the criteria for making judgments.
Moreover, the power law method serves to maintain the diversity that underlies human society. In human society, we have what is called a "long tail" property, which corresponds to the notion of the power law. Until recently, a business that deals with artworks such as movies and music, was considered to be successful if it produced a major hit. However, charts composed only of big hits do not form a power law. It is known that their sales are certain to fall at some point. As an example, in the case of a digital juke box containing 10,000 pieces of music offered via broadband for three months, it was reported that 98% of the pieces were accessed by users. This shows how vastly varied people's tastes are. Evidence of such phenomena can be observed even more clearly in situations such as online shopping. Online shopping does not just provide popular items: it is known that the more varied products an online shopping website offers, the more successful its sales will be. This shows how a small number of users always want something specific, but that there are many such small users, and the accumulation of these users is an important factor.
What exactly are you going to be doing in this project?
In brief, we are dealing with two themes - a technical study, and social implementation. In the technical study we will first analyze the performance of AI to see if a power law is formed. Generally speaking, AI does not form a power law. Even when there seems to be a power law, there are often crucial differences from human behavior. Given these results, we are interested in improving the current mathematical models underlying AI, so that their outputs satisfy power laws. As far as social implementation is concerned, we will try to consider the problems in AI investment and propose new social norms that can serve to maintain sound markets.
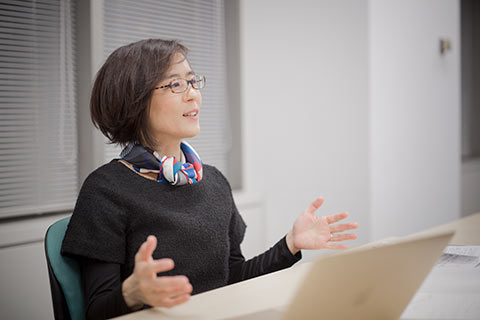
What problems lie in AI investment behavior?
In May 2017, the Financial Instruments and Exchange Act was amended in Japan in order to better accommodate AI in markets. However, the amendment only focused on keeping logs and submitting these records to the Financial Services Agency in the event that trouble arises. This would be nothing more than a response to something that had already happened. If a market crash similar in scale to Black Monday should occur as a result of AI, even if we analyzed the cause of such an event afterwards, it would be too late to avoid the huge impact. This is why we need measures to prevent such an event from occurring, or at the very least to stop it while it is happening. For this reason, we are discussing how to assess AI behavior with the help of power laws by detecting the moment when the power law stops holding. I hope this project will be a good start to considering questions such as "How can we stop AI that is motivated purely by profit?", and "How can we design evaluation methods to prevent such behavior?"
I would like to offer a new dimension to maintain the soundness of our society by assessing AI through power laws in order to help people working in both economic and other fields.
*Basic studies have been developed since 2014 by the PRESTO team of JST Strategic Basic Research Programs led to this project.
Date of interview: November 14, 2017
This interview has been printed in our Program Introduction booklet Vol.02. If you would like to check the other articles please click the link below.
Human Information Technology Ecosystem (HITE) Program Introduction booklet - Vol.01 Vol.02