Progress Report
Towards overcoming disorders linked to dementia based on a comprehensive understanding of multiorgan network3. Elucidation of Inter-Organ Networks by AI and Mathematical Research
Progress until FY2022
1. Outline of the project
Our aim is to understand inter-organ networks by constructing data-driven mathematical models in animal models combined with human data. In the data linkage system, AI and machine learning techniques will be used to make effective use of the limited data we have collected from living organisms, which enable us to remove noise from the data and fill in missing values.
By integrating mathematical models with human data, we will develop machine learning algorithms that can estimate the condition of each organ and identify the early stages of dementia using non-invasive and cost-effective measurements in the preclinical ‘ME-BYO’ conditions.
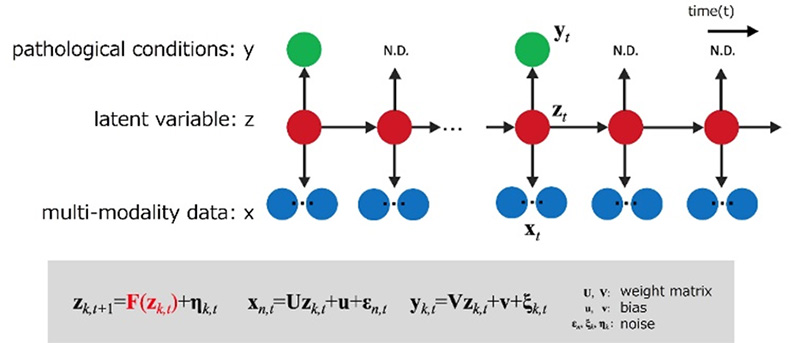
2. Outcome so far
(1) Deep Input-Output Stable dynamics (DIOS), a deep learning technique for learning dynamics from data
Kojima et al. at Kyoto University have developed a novel deep learning technique to learn the dynamics of biological motion from data while maintaining input-output stability, an important property of biological motion. The study focused on input-output stable systems that are robust against unexpected stimuli and noise. We then realized a method for learning neural network systems to guarantee input-output stability.

(2) Development of the pseudo-time reconstruction method from non-time series data
The pseudo-time reconstruction method is a technique that estimates the growth and differentiation processes of individual cells. Although this method is usually applied to single-cell RNA-seq data, Nakaoka and his research team in Hokkaido Univ. has developed a method to apply this technique to gut microbiome data.
Recently, the relationship between central nervous system disorders including Parkinson's disease-related dementia and gut bacteria has been attracting attention (gut-brain interaction). They plan to utilize their developed method to analyze data on gut bacteria in preclinical ‘ME-BYO’ individuals to identify gut bacteria associated with the onset and progression of disease based on gut-brain interaction.
In addition, a mathematical analysis called dynamic network biomarker (DNB) theory was applied to identify factors that can be used to identify conditions before the onset of the disease. As a result, they succeeded in detecting predictors before the changes of composition of the gut microbiota in attention-deficit/hyperactivity disorder and autism spectrum disorder. They will conduct further research to apply DNB-based analysis not only to gut microbiota data, but also to data related to dementia more broadly.
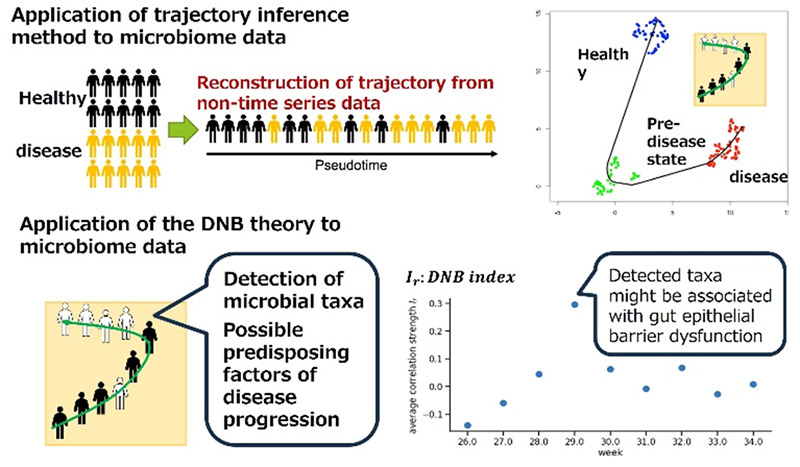
3. Future plans
we will construct data-driven mathematical models for multiple parameters obtained from animal models and preclinical human ‘ME-BYO’ cohorts to elucidate the transformation of the inter-organ network. We will also apply this to the achievement of prediction and prevention methods for dementia based on brain-organ interactions.