Research Results
A method for analyzing protein feature at a large scale
Development of a method for quantifying folding stability of a massive number of proteinsFY2024
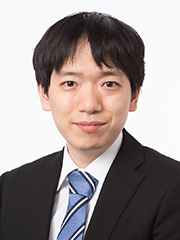
- TSUBOYAMA Kotaro (Lecturer, Institute of Industrial Science, The University of Tokyo)
- PRESTO
- Researcher (2022-2024) “Flexible disassembly and decomposition of higher-order structures using artificial proteins”
Measuring the folding stability of approximately 900,000 proteins in a single experiment
TSUBOYAMA Kotaro, a lecturer at the Institute of Industrial Science, The University of Tokyo (previously a postdoc at Northwestern University), has developed a method for measuring the folding stability of proteins in a high-throughput manner.
The folding stability of a protein generally indicates the proportion of a protein in its functional native structure. When the folding stability of a protein becomes lower, the proportion of the functional protein also becomes lower. Thus, many of the mutations that reduce folding stability cause various diseases because they reduce the amount of functional protein inside of cells.
Previously, researchers could only measure the folding stability of one protein at a time in a single experiment. Therefore, collecting folding stability data in the past required a lot of time, cost and effort.
In this study, the research group succeeded in measuring the folding stability of around 900,000 proteins at once by first converting the amino acid sequence information of proteins into DNA sequence information combined with a technique to rapidly decode DNA sequences. They then performed experiments where all of these proteins were exposed to protein cleaving enzymes at the same time, allowing them to accurately infer folding stability from the cleavage rate of these proteins using an algorithm that they constructed.
In recent years, there have been remarkable advances in AI technology based on deep learning models*1 such as ChatGPT, and many AI models for predicting protein properties have also been constructed, but they need a vast amount of data. However, even the largest database, combining almost all of the folding stability data published until now includes only ~30,000 stabilities. Using the new measurement method, they can now obtain data several times larger than the data accumulated so far, with only a single experiment (Fig.1). This new large scale data on protein folding stability is expected to be used in the development of AI models that can assist in the identification of amino acid mutations that cause various diseases, and in the efficient design of protein-based drugs.
*1 Deep learning models
Machine learning models based on structures known as a neural networks, which mimic the network of neurons in the brain. By making complicated multi-layer networks, it is possible to solve a variety of problems, such as the game of Go, with high precision.
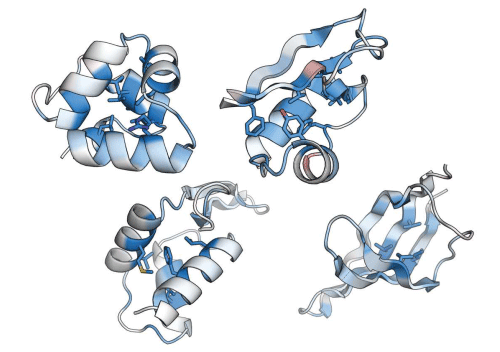
Fig.1 Visualization of the important sites for folding stability in proteins
These figures show the importance of each site for folding stability in proteins (darker blue/red indicates more/less importance). To produce even a single figure, we need to measure the folding stability of 1000-2000 amino acid sequences, making it difficult to visualize such folding stability using conventional folding stability measurement methods.
Measuring protein folding stability is challenging due to time and cost.
Proteins play a critical role in regulating various biological functions, and are essential for living things.
Almost all proteins switch between multiple states, including unfolded, folded and intermediate states. Typically, proteins are functional only in the (properly) folded state. The folding stability of a protein, which indicates the proportion of the protein in its folded state, usually indicates the proportion of the protein in its functional form. Therefore, the folding stability of a protein is an important feature to understand how the protein functions.
However, since there are not enough data about the folding stability of proteins, our understanding of protein folding stability is largely limited.
This is primarily because we could not measure the stability of a massive number of proteins simultaneously. Instead, each protein requires independent measurements, which requires a significant amount of time and effort.
The development of a method for efficiently quantifying the folding stability of proteins
The research group applied two techniques in their method for efficiently measuring the folding stability of proteins.
The first technique is a method to read out the amino acid sequence information of proteins. In recent years, with the development of next-generation DNA sequencing*2, it has become possible to more accurately analyze a larger number of amino acid sequences by reading out the DNA sequences rather than by reading out the amino acid sequences directly. Thus, cDNA display *3 is used to link each protein to its corresponding cDNA. Combining the two technologies of cDNA display and next-generation DNA sequencing, the research group could analyze an extremely large number of proteins in a single experiment.
The second technique is using protein cleavage enzyme, protease, for quantitation of the folding stability of proteins. Protease can efficiently cleave proteins in an unfolded state, but not those in a folded state. Utilizing this property of protease, the researcher could accurately quantify the folding stability of a protein by measuring the rate at which it is cleaved.
By combining these methods, the research team successfully quantified the folding stabilities of ~900,000 proteins in a single experiment.
Using this new method, the research team investigated the mechanism of how ~500 natural and designed proteins can keep their tertiary structures.
To investigate which amino acid sites of the proteins are critical for maintaining their structure, the folding stability was measured for amino acid sequences with amino acid replacement, insertion (Ala/Gly), and deletion for each site in each domain. This allowed them to visualize which sites are important for maintaining structure of the proteins (Fig.2).
*2 Next-generation DNA sequencing
A technology enabling identification of sequences of up to about 10 billion of DNA sequences in a single analysis. Since it can accurately count each DNA sequence, in combination with cDNA display *3, researchers can quantify the amount of a large number of proteins.
*3 cDNA display
A method for linking proteins to corresponding cDNA in a test tube. By decoding the cDNA sequences, it is possible to identify the amino acid sequence for the proteins.
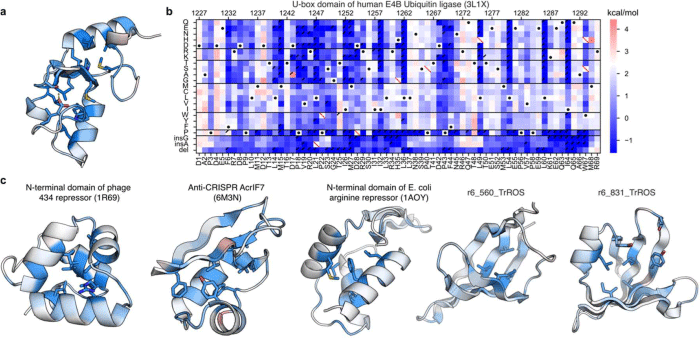
Fig.2 Structure diagrams of the folding stability of proteins
(a,c) These pictures show the importance of each location in the protein for its folding stability (darker blue indicates greater importance and darker red indicates less importance).
(b) The source data for the protein figure in (a). The heatmap shows the folding stability of the wild-type protein (white/black dots) and amino acid replacements/inserts/deletions where the folding stability decreased (blue) or increased compared to the wild type. To create a structure diagram for a protein, it is necessary to measure the folding stability of a thousand or more amino acid sequences. The horizontal axis shows the location within the protein and the vertical axis indicates the substitution, deletion, or insertion of amino acids.
The present study will serve as a foundation for the next generation of protein science
In recent years, protein research has been shifting from hypothesis-based studies to acquiring and analyzing large-scale data to understand protein properties and functions. This has led to the elucidation of characteristics and functions for a broad range of proteins. Such new protein research requires both large-scale data and advanced analytical techniques.
The present study contributes greatly to large-scale data collection of proteins. This can be expected to lead to the development of AI models for predicting amino acid mutations of proteins that can cause disease and for efficiently designing next-generation protein drugs.
- Life Science
- Research Results
- Japanese