Research Results
Accelerating Life Sciences Research
Culturing iPS Cells through Collaboration between
Humans, Robots and AIFY2023
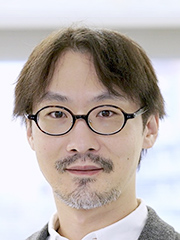
- TAKAHASHI Koichi (Team Leader, RIKEN Center for Biosystems Dynamics Research)
- JST-Mirai Program
- “Common Platform Technology, Facilities, and Equipment” mission area, "Accelerating life sciences by robotic biology", Project Leader
(Feasibility Study: 2018-2020, Full-scale R&D: 2021-2025)
Automation technology for producing cells for the retina of the eye from iPS cells
This research group has developed a robot/AI system that automatically performs trial-and-error to examine the optimum conditions for culturing iPS cells, and has succeeded in improving the recipe for cell culture used in actual regenerative medicine.
A system combining the versatile humanoid robot LabDroid "Maholo"*1 (Fig. 1), which is capable of conducting high-precision life science experiments, and newly-developed artificial intelligence (AI) software (optimization algorithm) was designed. In the process which induces differentiation of iPS cells (induced pluripotent stem cells)*2 into retinal pigment epithelial cells (RPE cells)*3, whereby the cells become more complex and heterogeneous, it was demonstrated that this system was proved to autonomously discover efficient culture conditions without human intervention.
*1 Versatile humanoid robot LabDroid "Maholo".
Humanoid robot system for life science experiments developed by Robotic Biology Institute Inc. (a subsidiary of Yaskawa Electric Corporation.) The same laboratory equipment used by humans in experiments is arranged around an industrial, 7-axis dual-arm robot of Yaskawa Electric Corporation. Experimental operations that were previously carried out by hand by humans, such as manipulating a pipette (a generic term for a relatively simple plastic tool that accurately separates a certain volume of liquid and transfers it to another container), and opening and closing the door of an incubator (a device that maintains a constant temperature), can now be performed by the robot.
*2 iPS cells (induced pluripotent stem cells)
Pluripotent stem cells (undifferentiated stem cells that can differentiate into all tissue cells and germ cells that make up the human body), artificially created by introducing genes such as Oct3, Sox2 and Klf4 into somatic cells such as adult skin cells, to make them pluripotent.
*3 Retinal pigment epithelial cells (RPE cells).
One of the cell types that make up the retina. Clinical research on regenerative medicine using iPS cell-derived RPE (retinal pigment epithelium) cells is being undertaken by RIKEN, Kobe City Eye Hospital, and other institutions.
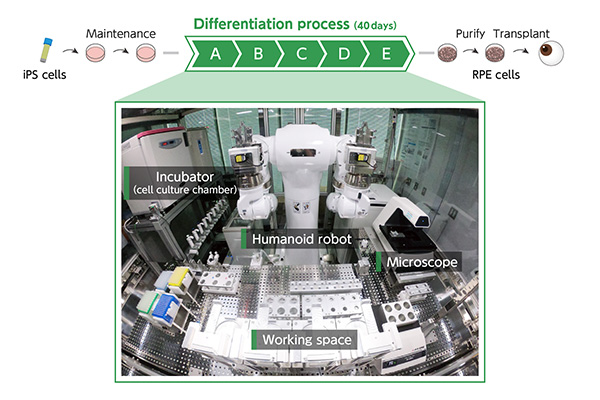
Fig. 1 LabDroid "Maholo".
Implemented process of differentiation from iPS cells into RPE cells.
Technical issues specific to experiments with cells
Science is the activity of expanding the wisdom of mankind. Scientific research activities involve a large number of experiments which are designed to screen a large number of conditions, and then implement them in the real world. The conditions include a wide range of parameters (reaction time, reagent concentrations, operating sequence, etc.) required to carry out an experiment, which necessitates a very long period of trial and error. Autonomous experiments, in which scientific experiments are carried out autonomously without human intervention, are currently being promoted in various fields as an effort to reduce the trial-and-error burden on researchers.
Successful examples of autonomous experiments have emerged in several fields, such as 'composition ratios of photocatalytic mixtures used in fuel cells' and 'genetic sequences of microorganisms to improve production efficiency, targeting material production by microorganisms'. However, there have been no examples of implementation in the fields of regenerative medicine and cell biology, as typified by the differentiation of iPS cells. This is because of two technical barriers to cell culture - the fact that minute differences in experimental technique have a significant impact on quality, and the fact that the differentiation of iPS requires a long culture period (several weeks to several months).
Demonstration of autonomous experiment in the field of cell culture
This study addressed the demonstration of an autonomous experiment in the field of cell culture, in three steps:
[Step 1 / Implementation of protocols by robot], [Step 2 / Determination of autonomous experiment strategy] and [Step 3 / Demonstration experiment].
[Step 1 / Implementation of protocols by robot]
The entire process of transplanting RPE cells differentiated from iPS cells into patients was broken down into five steps A to E, and the procedures required for each step were implemented by the versatile humanoid robot LabDroid, 'Maholo'. Maholo was designed to handle eight 6-well (hole) plates per experiment, and to perform cell culture in a total of 48 wells simultaneously. The actual culture results showed that the required protocols could be successfully implemented by the robot, as colored cells indicating differentiated RPE cells (pre-differentiated iPS cells are colorless, and differentiated RPE cells are brown), appeared in all 48 wells.
When the differentiation efficiency was evaluated in terms of colored cells, the percentage of colored cells in the wells was around 40-50%, indicating that the efficiency of differentiation was insufficient and further improvement was required (Fig. 2). The culture conditions and procedure in Step 1 were the same as those for ordinary human manual work (standard conditions), suggesting that the optimum conditions for robots and humans were actually different. It was therefore attempted to improve the differentiation efficiency when the robot was culturing the cells.
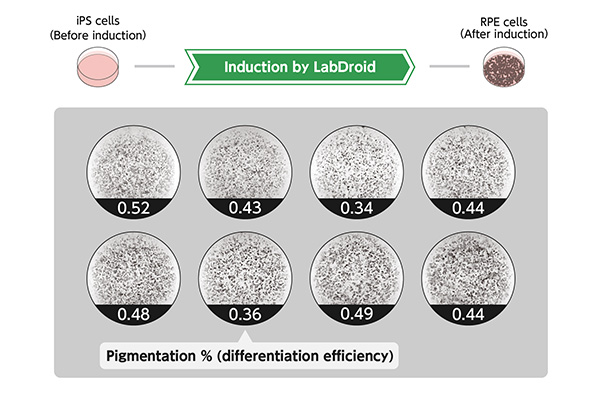
Fig. 2 Differentiation efficiency of RPE cells using the LabDroid, 'Maholo' (colored state).
Differentiation results using the LabDroid 'Maholo' under the same conditions as those of ordinary human manual work (standard conditions). The black areas are RPE cells considered to have been correctly differentiated, and white areas are others. About 40-50% coloration was observed under all conditions.
[Step 2 / Determination of autonomous experimental strategy]
In the process of differentiation of iPS cells to RPE cells, there are numerical values (parameters) that must be set appropriately, such as the concentration of reagents and the length of cell processing time. In this study, the strategy for deriving a combination of parameters that improves the differentiation efficiency was formulated (modeled) as a black box optimization problem*4, and a specific method was selected. Bayesian optimization*5, a commonly used algorithm for solving the black box optimization problem, was used as the optimization method for the autonomous experiments. However, general Bayesian optimization requires many rounds of experimentation, evaluation and planning to be repeated, one condition at a time, and when applied to the differentiation of iPS cells to RPE cells, each round requires 40 days of culture, so batch Bayesian optimization*5, an extended form of Bayesian optimization, was adopted. Batch Bayesian optimization shortens the experimental period by introducing multiple conditions per round, and in this study, three rounds of 48-condition experiments, 48-condition evaluations and 48-condition planning were conducted.
The parameters to be varied (screening range) and the values to be maximized (evaluation values), which are necessary to apply batch Bayesian optimization, were defined. Based on expert advice and computer simulations, seven of the processes required for differentiation of iPS cells to RPE cells were defined as parameters (Fig. 3). To indicate the differentiation efficiency, the colored cell rate was used. Based on the above, artificial intelligence (AI) software (an optimization algorithm) capable of implementing the strategy was developed, and then tested (Fig. 4).
*4 Black box optimization problem
In cell culture, it is difficult to intuitively derive which parameters should be changed and how (inputs), in order to improve the differentiation efficiency (outputs), due to the complexity of the internal state of the cells. This situation where the relationship between inputs and outputs is unknown is called a 'black box', and the problem of finding the inputs that maximize/minimize the black box outputs through trial and error is called a black box optimization problem.
*5 Bayesian optimization and batch Bayesian optimization
Bayesian optimization is an algorithm that balances the selection of conditions that are predicted to be good (utilization), and unknown conditions that have not yet been tested (exploration), based on the results of previous experiments. It is used to find the maximum or minimum value of a function whose shape is unknown (black box function).
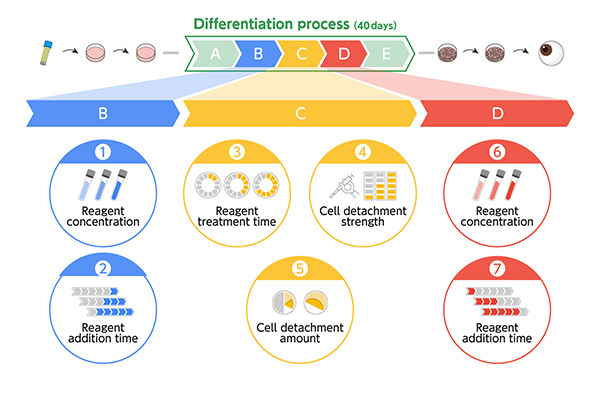
Fig. 3 Parameters to be screened
[Step 3 / Demonstration experiment]
The research group conducted actual experiments using the LabDroid 'Maholo' on the 48 conditions derived by batch Bayesian optimization, and evaluated the differentiation efficiency (colored cell rate) for each condition. While using the optimization algorithm, the experimental design, experimental implementation, evaluation and prediction were repeated until Round 3 to search for the optimum parameters (Fig. 4). As a result of these experiments, the highest evaluation value was successfully obtained in Round 3 (Fig. 5). A final evaluation test was also conducted, which confirmed a statistically significant improvement in the evaluation values compared to the conditions before screening by the optimization algorithm.
These results show that the system combining the versatile humanoid robot LabDroid 'Maholo' and the optimization algorithm enables autonomous experiments in the field of cell culture to discover culture conditions that increase the efficiency of differentiation of iPS cells to RPE cells, without human intervention.
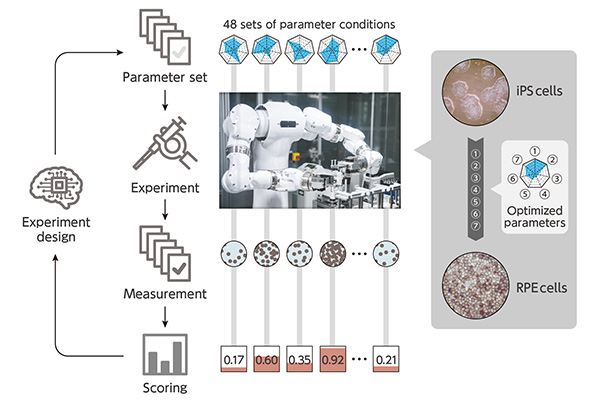
Fig. 4 Autonomous experimentation strategies and workflow
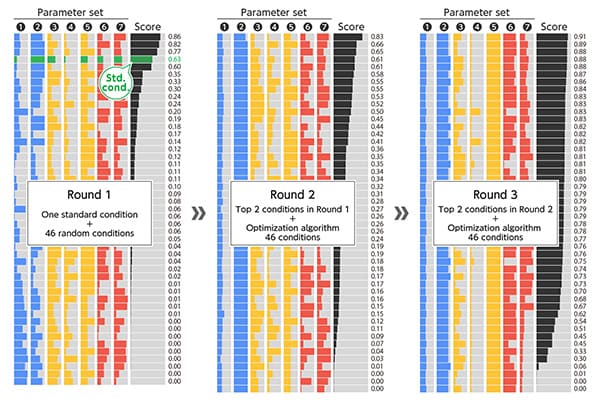
Fig. 5 Results of demonstration experiment
Parameter sets (experimental conditions) and evaluation values (colored cell rate) in Rounds 1-3. Green in Round 1 indicates standard conditions. The highest evaluation value (91%) was obtained in Round 3.
Contributing to the acceleration of life sciences research
Although this research was conducted in the field of cell culture, it is expected that the combination of the LabDroid 'Maholo' and AI systems can be applied to a wide range of fields. If autonomous execution of trial-and-error in many life science experiments becomes commonplace, the progress of life science research is expected to be greatly accelerated.
The automation of life science experiments will also open up new partnerships between humans and robots/AIs. In the system in this study, the roles are divided between humans with expertise in defining the target process, screening range and evaluation values, while the robot/AI is responsible for the trial-and-error experiments. This division of labor allows researchers to concentrate on intellectual creativity.
- Life Science
- Research Results
- Japanese