Progress Report
Construction of an AIoT-based universal emotional state space and evaluation of well-/ill-being states[1] Constructing a Human Emotional State Space by AIoT
Progress until FY2023
1. Outline of the project
In this project, we aim to develop AIoT (AI×IoT) technology that enables the objective estimation of emotional states in daily life on a cloud platform using multidimensional psycho-physiological data (e.g., voice data, physical activity, heart rate, respiratory rate, and recording situations) measured by IoT devices. The project is divided into the following three research topics:
- 1. Development of human emotion estimation technology with clinical validity using multidimensional psycho-physiological data
- Summary: we develop technology with clinical validity that enables the objective estimation of various emotional states on a cloud platform. This is achieved by utilizing multidimensional psycho-physiological data (e.g., voice data, physical activity, heart rate, and respiratory rate) measured by IoT devices in daily life.
- 2. Assessment of psycho-physiological data in patients
- Summary: we collect psycho-physiological data from patients with mental disorders to construct a human emotional state space with clinical validity.
- 3. Development of a translational IoT cloud system
- Summary: we develop a cloud-based IoT system capable of acquiring continuous and real-time psycho-physiological data from both humans and animals (mice and rats) in real-world settings on a large scale.
2. Outcome so far
To develop human emotion estimation technology, we constructed machine learning models that estimate self-reported emotion scores recorded in daily life from simultaneously measured physiological signals using our existing database (Figure 1).
[Main Achievements by FY2022]
- Estimated scores for four emotions (depressed mood, anxiety, positive mood, and negative mood) from spontaneous physical activity data with an error margin of approximately 20%.
- Estimated nine emotions (“vigorous,” “gloomy,” “concerned,” “happy,” “unpleasant,” “anxious,” “cheerful,” “depressed,” and “worried”) from voice data with an average concordance correlation coefficient of 0.55 and a maximum of 0.61.
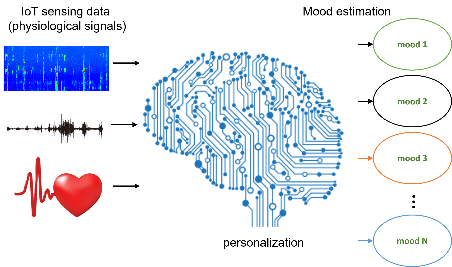
[Main Achievements in FY2023]
By introducing a personalized approach for the binary classification of the levels (high/low) of four emotions (depressed mood, positive mood, negative mood, anxiety) based on physical activity, we achieved an estimation accuracy exceeding 70% in terms of accuracy, recall, precision, and F1-score. Additionally, using a machine learning model combining a different personalized approach, we estimated subjective scores for nine emotions (“vigorous,” “gloomy,” “concerned,” “happy,” “unpleasant,” “anxious,” “cheerful,” “depressed,” and “worried”) from physical activity data with an average concordance correlation coefficient of 0.48. Furthermore, we successfully improved the estimation accuracy by constructing multimodal machine learning models that utilize multiple physiological signals (voice and physical activity).
To construct a translational IoT cloud system for humans and animals, we have been developing and implementing a ring-type device aimed at "subtle" sensing. In FY2023, we completed the integration of the device with the existing IoT cloud system. Additionally, we conducted a proof of concept (PoC) study for monitoring vital signs, stress, and sleep in residents of a dementia floor in a nursing home, with the aim of verifying the system and expanding it to service businesses (Figure 2).
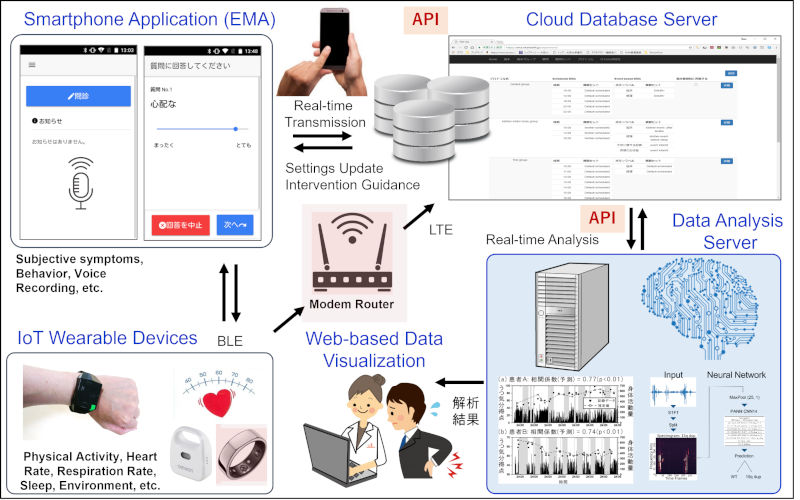
As an extension of this system to animal measurements, we created and validated a prototype sensor for mice.
3. Future plans
- We will continue to collect clinical data (from patients with mood disorders, anxiety disorders, and alexithymia) and use them to develop emotion estimation models with clinical validity.
- We plan to develop animal devices for animal IoT sensing.
(NAKAMURA Toru: Osaka University
YAMAMOTO Yoshiharu: The University of Tokyo
YOSHIUCHI Kazuhiro: The University of Tokyo)