Progress Report
AI & Robots that Harmonize with Humans to Create Knowledge and Cross Its Borders[1] Experiment Automation AI Robot
Progress until the fiscal year 2023
1. Overview
We are developing an AI robot that, after conceiving experiments based on research hypotheses, estimates specific procedures in cyberspace and executes them in physical space.
Specifically, we are conducting R&D on (1) "AI for understanding experimental papers" to plan experiments from past cases, (2) "Exploration of AI robots performing organic synthesis" to conduct automated experiments, and (3) "XOR discovery AI for experimental predictions and results" to verify hypotheses from the outcomes.
2. Progress
This project sets the realization of AI that understands human research through scientific literature as its first milestone.
For the experiment automation AI robot, it is necessary to first plan experiments from related case studies in papers, as researchers would when replicating existing studies, and estimate and execute specific experimental parameters. However, since literature often only outlines experimental settings, this project attempted to infer by collecting knowledge from literature on different topics as well.
Additionally, it is necessary to examine results from experimental graphs to verify hypotheses. Thus, this project is developing AI models that can provide insights on experimental result figures in papers.
(1) AI for Understanding Experimental Papers
To understand and compare experimental content, we worked on "semantic analysis of tables" to extract and structure information such as tasks, data, and methods listed in paper tables. We input text-related information from tables into large language models (LLMs) to generate auxiliary explanatory text called synthetic context. Using this synthetic context as a feature for machine learning models improved entity linking accuracy by over 5 points compared to conventional methods. Moreover, utilizing text from cited literature complemented auxiliary knowledge not described in the paper itself, contributing to improved linking accuracy.
We also collected and annotated data on synthesis procedures targeting materials science literature, confirming that an initial BERT-based model could estimate synthesis procedures.
(2) AI Robots Performing Organic Synthesis
To represent compounds, we constructed a network-type database (Molecular Reaction Graph) with synthesis paths as edges and molecules as nodes, and fabricated a Kyoto University-style automated synthesis device, simplifying "Chemputor" (Fig. 1). Aiming for automatic input of experimental procedures, we approached the realization of an automatic generation program by converting experimental procedure text to Mermaid notation using ChatGPT. We successfully conducted 0.3 mol esterification, acetalization, and amidation experiments. Future work will address issues with post-processing and cleaning of experiments using deleterious and toxic substances.
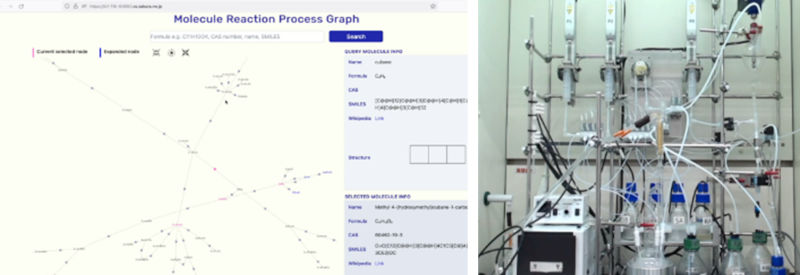
(3) XOR Discovery AI for Experiments
First, we built an AI that understands and explains figures in papers, developing a reliable AI incorporating researchers' insights. As existing models struggle with detailed explanations, we explored methods to input researcher-emphasized areas into the model. By manipulating Attention Weights in the Self-Attention mechanism, we generated captions detailing emphasized regions. Experiments confirmed the generation of captions containing words related to emphasized areas (Fig. 2).
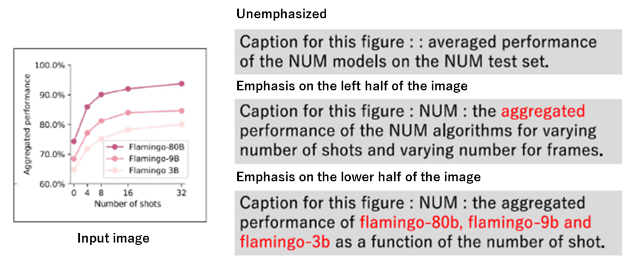
3. Future work
The milestone up to fiscal year 2025 includes not only continuing to understand research but also realizing hypothesis generation. Physical space experiments are crucial for this, and research acceleration is necessary to prevent this from becoming a bottleneck.
Specifically, we will first expand the exploration space of target substances by enhancing automated synthesis methods. We will simultaneously explore diverse synthesis methods such as flow synthesis and mechanochemical synthesis.
We also plan to incorporate synthesis execution while updating initial hypotheses for candidate substances to those easier to synthesize or more likely to react, considering hypothesis updates utilizing simulations.
Lastly, synthesizing such candidate substances requires estimating synthesis routes and synthesis conditions.
Thus, this project aims to increase the throughput of the research loop by further advancing the experiment automation AI robot.