Progress Report
Co-evolution of Human and AI-Robots to Expand Science Frontiers2. Brain of the AI-robot scientists
Progress until FY2022
1. Outline of the project
The brains of AI-robot scientists, which autonomously explore science, require intelligence to plan actions on the target, observe its reaction, and update the plan. If this update can be performed autonomously, then the AI-robot scientists can enhance their intelligence through autonomous interaction with the environment.
In the project, it is assumed that the brains of AI-robot scientists can be developed by integrating (1) AI for Knowledge, which handles explicit data to interpret experimental results and propose hypotheses, (2) AI for Skills, which handles implicit data to interpret experimental manipulations and propose manipulation strategies, and (3) Mathematical Foundations, which abstract knowledge and skills to structure them as intelligence.

2. Outcome so far
Component technologies have been developed for experiments conducted by collaborating scientists.
(1) AI for Knowledge (Takeuchi-PI, Mori-PI)
The AI for Knowledge is under development to interpret experimental data, acquire new knowledge, and formulate hypotheses, taking into account processing capabilities. It focuses on explicit knowledge. For instance, an autonomous AI system is currently being developed to generate potential compound hypotheses by utilizing various sources of knowledge, such as compound structures, literature, and previous experimental results. Additionally, an advanced image-processing AI has been designed to automatically analyze experimental data, enabling higher-level interpretation. The automatic quantification of microstructure features in microscopic images enables the acquisition of insights and knowledge that surpass the measurements conducted by scientists.
(2) AI for Skills (Taniguchi-PI, Okada-PI)
The AI for Skills is designed to learn skills from observed experimental manipulations and formulate manipulation strategies while considering the robot's own physical capabilities. This methodology specifically focuses on implicit skills. In the future, AI for Skill will have the ability to develop strategies for configuring the physical structure of AI-robot scientists (Self-Reconfiguration), allowing for manipulation strategies that are unique to AI robots. As a result, this will enable the realization of experimental manipulations that are distinct from those performed by human scientists.
AI methods are currently being developed to utilize various cues, such as images, sound, and vibrations, in order to learn the interactions between robots and their targets. Through this, the AI can uncover the characteristics of these interactions and form a latent state space. An example of this is the development of robot control based on Multimodal NewtonianVAE (Fig. 1). Additionally, a method has been devised to learn skilled operations through tele-robotic manipulations and autonomously perform tasks using a limited amount of training data.
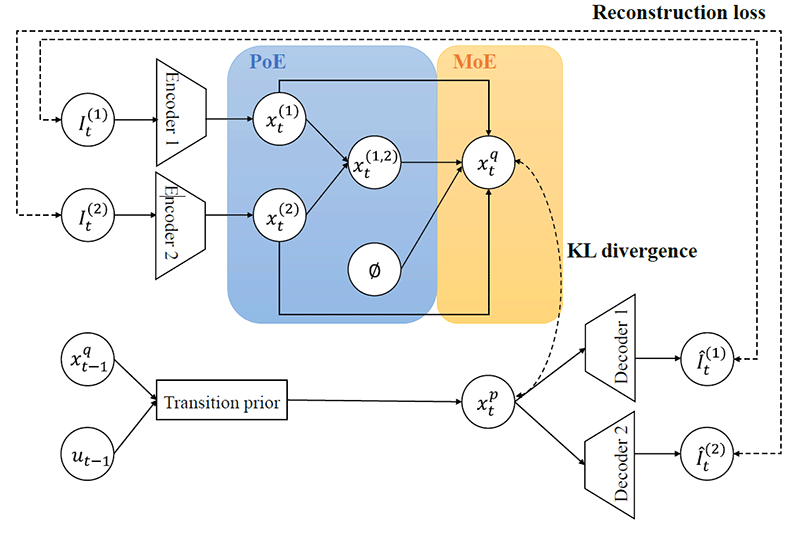
(3) Mathematical foundations (Tanimura-PI, Maruyama-PI, Matsubara-PI)
Scientific exploration is to correlate various data and find a systematic structure in it. The AI for Knowledge and Skills is to search in vast latent spaces, and providing Mathematical Foundations to the AI is to correlate the data in the latest spaces to abstract it as intelligence.
The abstract mathematics called category theory is being studied, and conventional neural architectures were successfully expressed using the category theory. Methodologies to incorporate existing principles or discover new principles in the vast latent spaces are also studied.
3. Future plans
The AI technologies will be integrated to the robotic bodies for enabling autonomous scientific exploration.